Colloidal Synthesis: The ultimate goal of QD synthesis is to obtain a sample of monodisperse (all perfectly identical) QDs of a specific size. Unfortunately, such procedures do not yet exist and so we aim to produce QD samples with very low size-dispersity (almost identical). A second objective is to produce QDs with near unity photoluminescence quantum yield—all absorbed photons are reemitted. While these two first goals have generally been met, a third objective aims to develop synthetic routes that yields QDs that are stable in working devices. To combine these objectives we need to develop elaborate synthetic schemes.
We will primarily make use of the hot injection method developed by Murray, Norris and Bawendi and published in their seminal 1993 paper. This method involves the injection of a precursor, let’s say a trisdimethylamido-phosphine, to a heated solution containing the complementary precursor, let’s say indium chloride, to produce very low size distribution ensembles of QDs, such as InP QDs. This method has been extended to all QD compositions studied within the network.
The hot injection method can be divided into four key steps:
- Preparation of Precursors: metal precursors (cadmium oleate, indium chloride, copper acetate, …) and chalcogenide or pnictogen precursor (trioctylphosphine-sulfide, trisdimethylamido-phosphine, …)
- Rapid Injection: The precursor solution, usually the chalcogenide or pnictogen, is swiftly injected into a hot solvent containing the metal precursors. This triggers nucleation.
- Crystal Growth: The presence of nuclei leads to QD growth. By controlling variables such as reaction time, temperature, and ligand composition, the size and shape can be controlled.
- Purification: The synthesized QDs are purified through an antisolvent based purification. These QDs are then ready for post-processing.
To perform our reaction we will make use of a Schlenk line which ensures an oxygen and moisture free reaction environment.
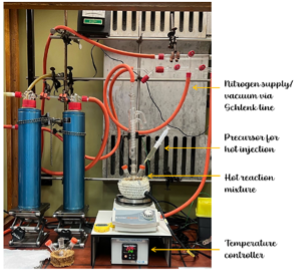
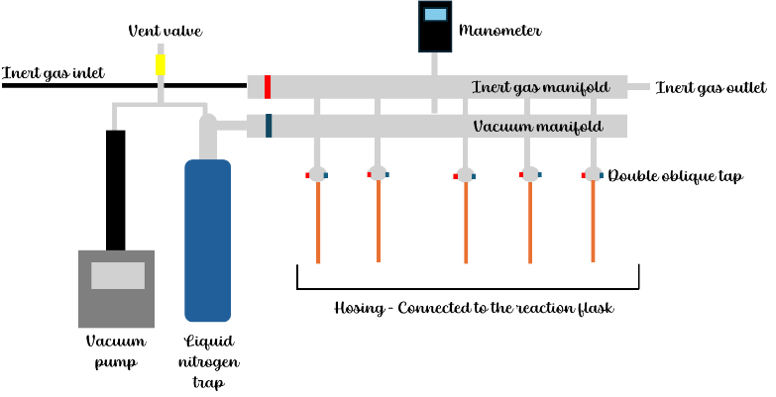
Figure: Our Schlenk line setup for QD synthesis
Our 'Track the Twin' research group will leverage the hot injection method to synthesize state-of-the-art quantum dots for advanced applications. By refining synthesis parameters and exploring novel precursors with the help of the “Digital twin”, we aim to develop next-generation QDs with superior optoelectronic properties and profound resilience against loading-induced aging. Our aim is to obtain QDs with:
• uniform size and shape
• narrow photoluminescence linewidths
• enhanced stability
Throughout the project, we will test various synthetic conditions and reveal mechanistic insight to synthesize optimized QDs. We will also make use of high-throughput methods to accelerate our search of ageing-resistant QDs.
By Aishwarya B Narayana
Automated Synthesis: In the Track The Twin project, we envision using automated tools for the preparation of selected colloidal semiconductor core/shell quantum dots and bulk nanocrystals to facilitate the screening of synthesis conditions and accelerate their precise structural engineering. We aim to transfer synthesis protocols developed on the bench to enhance reproducibility by our TTT network, reducing the manual effort required by conventional approaches. Through this automated exploration, we will also investigate the chemical tuning of non-toxic alternative precursors, and material scalability in volume to produce photostable structures.
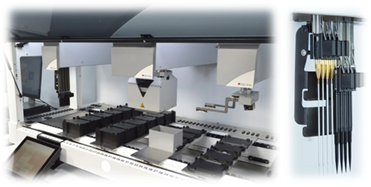
(4D) STEM: Scanning Transmission Electron Microscopy (STEM) is a high-resolution imaging technique that visualizes nanomaterials by scanning a focused electron beam across a sample. 4D-STEM advances this method by capturing a 2D diffraction pattern at each scan point, which provides richer data and minimizes beam damage making this technique particularly valuable for characterizing complex beam-sensitive materials such as quantum dots (QDs). By combining 4D-STEM imaging with precise TEM quantification analysis, researchers can now resolve and count individual atoms, providing unprecedent insights into QDs’ atomic structure, composition, and properties.
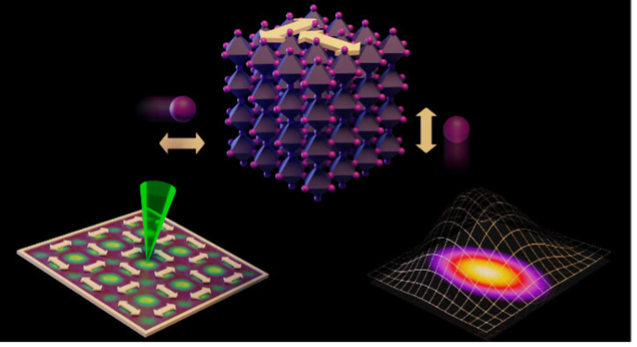
Image: Schematic illustration of the 4D-STEM technique to atomically resolve the structure of a FAPbBr3 quantum dot. DOI: 10.1021/acs.nanolett.4c02811
By: João Gabriel Gonçalves Chiquito
Solid-state NMR: Structural characterization on an atomic level is one of the fundamental challenges in modern chemistry. Knowledge of the arrangement of atoms in a material is a key to understanding its properties which in turn is crucial for the design of systems with tailored qualities. Solid-state nuclear magnetic resonance (ssNMR) spectroscopy is a powerful non-destructive analytical technique suitable for that task. Besides being nuclear specific, it is extremely sensitive to the topology and geometry of the surrounding of the observed nuclei, small changes in them or their distribution. NMR has no limitations concerning the crystallinity of the sample, which is crucial for analysing nanoscale materials, and is sensitive to potentially occurring structural disorder or dynamics. In this project, ssNMR will be employed to correlate the different chemical environments of elements observed in the spectra with the core-shell structure and surface termination of quantum dots, as well as to track structural changes in the material upon aging.
By Lidiia Dubenska
Small Angle Neutron Scattering (SANS) is a powerful technique used to study the structure and properties of materials at the nanometer scale. It involves the scattering of neutrons from a sample, with the angle of scattering being small. When neutrons interact with the atoms in the material, they are scattered in different directions, and by analyzing the intensity and angle of the scattered neutrons, researchers can gain insights into the sample’s internal structure, such as its size, shape, and arrangement of molecules or particles. SANS is particularly valuable for in-operando measurements of quantum dots, allowing the observation of their structural evolution and behavior under real-time conditions, such as during synthesis, device operation, or environmental changes, providing critical information for optimizing quantum dot-based technologies.
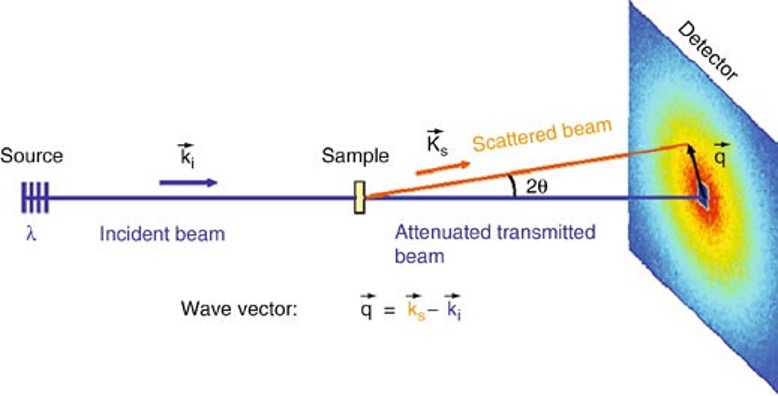
Image taken from Grillo, I. (2008). Small-Angle Neutron Scattering and Applications in Soft Condensed Matter. In: Borsali, R., Pecora, R. (eds) Soft Matter Characterization. Springer, Dordrecht. https://doi.org/10.1007/978-1-4020-4465-6_13
Single QD spectroscopy: Nanocrystals are typically made in large batches using chemical synthesis. Each nanocrystal in the batch is slightly different in terms of size, shape, and composition. This leads to variations in properties, which in turn affects the overall performance of the ensemble of nanocrystals. To guide the design and optimization of nanocrystals for various applications, we need to understand these variations directly. We perform experiments on individual nanocrystals, precisely recording for example the timing and wavelength of individual photons emitted. Such experiments are challenging, because the signal from an individual nanocrystal is very weak.
Ultrafast spectroscopy: Ultrafast spectroscopy is a time-resolved technique with sub-picoseconds time resolution, that is used to trace excited state dynamics; it allows us to see how excitons behave. The figure below shows the basic setup of ultrafast transient absorption (TA) spectroscopy measurement. A pump pulse is used to excite the sample. This is followed by second pulse which probes the excited sample. What we extract is the differential absorption (ΔA) between the steady-state absorption and the transient absorption after excitation. By tuning the delay time (τ) between the pump and probe pulses, we can trace the evolution of the excited states as a function of time.
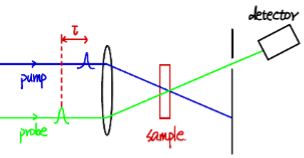
Figure: The schematic of ultrafast spectroscopy measurement.
By Chia-Kai Lin
Ultrafast electron diffraction is a state-of-the-art technique that enables the direct observation of structural dynamics in quantum dots (QDs) with femtosecond time resolution. By scattering ultrashort electron pulses off QDs, UED captures transient atomic rearrangements and lattice distortions following photoexcitation, offering a unique window into the ultrafast processes governing their behavior. This method is instrumental in elucidating carrier-induced structural changes, phase transitions, and electron-phonon interactions, which are critical for understanding the fundamental properties of QDs.
UED plays a pivotal role in revealing how QDs respond to light at ultrafast timescales, shedding light on non-equilibrium dynamics that influence their electronic and optical properties. Such insights are essential for optimizing QDs for next-generation applications in optoelectronics, quantum computing, and energy conversion technologies.
Experiments utilizing UED require specialized facilities equipped with high-brightness electron sources, ultrafast laser systems for precise photoexcitation, and advanced electron detectors capable of capturing structural changes with sub-picosecond resolution. Large-scale research centres host cutting-edge UED setups that provide the high temporal and spatial resolution needed to explore QD dynamics in unprecedented detail.
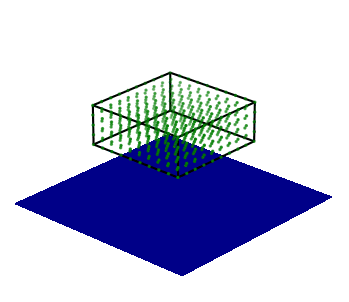
By Dogu Seyda
DFT : Density Functional Theory (DFT) is a computational method for calculating the electronic structure of materials by approximating the many-body Schrödinger equation. Since it is derived from quantum mechanical principles, it does not require empirical input parameters. In the context of quantum dots (QDs), accurately predicting their behavior under various conditions requires a detailed model of their structure, particularly the surface and surrounding ligands.
The primary goal of this part of the project is to leverage DFT calculations to generate a high-quality training set for developing machine learning force fields (MLFFs) for both the ground and excited states of QDs. A key objective is to strike an optimal balance between accuracy and computational efficiency during training while enabling the ML model to adapt dynamically. This adaptability is crucial for capturing the reactivity of photoactivated QDs with environmental species such as oxygen and water in real-time simulations
By Masuma Suleymanova
Machine-learning force-fields: One of the first applications of machine learning to the physical sciences has been in the design and application of Machine Learned Force Fields (MLFF).
MLFF are usually Graph Neural Networks (GNNs) trained on large datasets of energy and force evaluations with high accuracy ab initio methods. The GNN ``learns'' to express the complex relation between a configuration of atoms and its corresponding effective potential energy of the nuclei, in the Born-Oppenheimer approximation.
In this project, Professor Clementi is developing MLFF to capture ground and excited states of Quantum Dots (QDs). MLFF can aid in the analysis of QD surface reactivity through thermodynamic and kinetic components, and is foundational for constructing the QD digital twin toolbox.